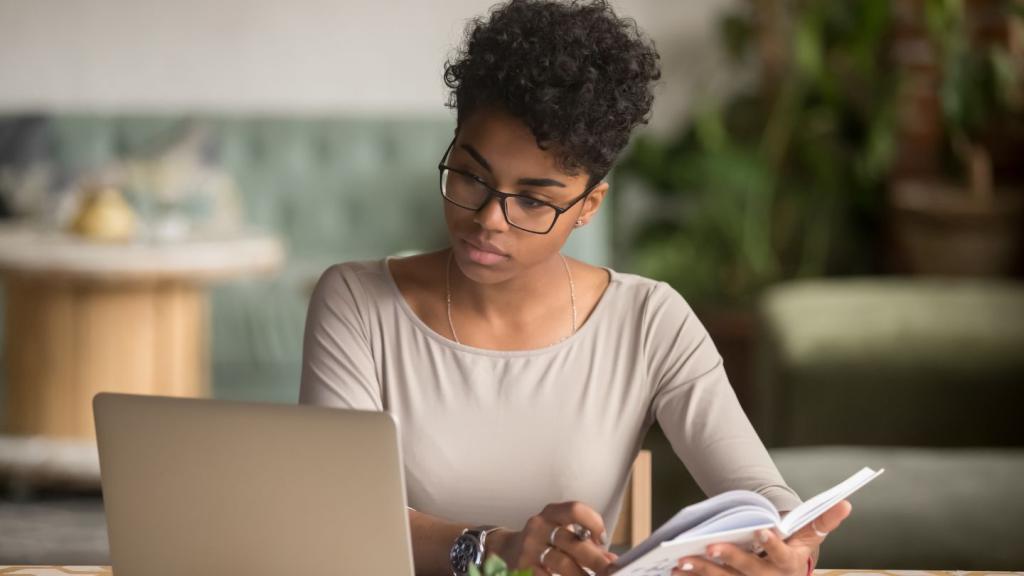
The more you understand about primary immunodeficiency (PI), the better you can live with the disease or support others in your life with PI. Learn more about PI, including the various diagnoses and treatment options.
Living with primary immunodeficiency (PI) can be challenging, but you’re not alone—many people with PI lead full and active lives. With the right support and resources, you can, too.
Be a hero for those with PI. Change lives by promoting primary immunodeficiency (PI) awareness and taking action in your community through advocacy, donating, volunteering, or fundraising.
Whether you’re a clinician, researcher, or an individual with primary immunodeficiency (PI), IDF has resources to help you advance the field. Get details on surveys, grants, and clinical trials.
In recent years, machine learning has jumped from science fiction into everyday life—voice assistants, translation apps, and fraud detection software all use machine learning technology. In healthcare, machine learning has been used to detect potential tumors on radiology images and transcribe clinical notes into electronic health records. Diagnosing conditions like primary immunodeficiency (PI) earlier and more accurately is next on the horizon.
Machine learning is a kind of artificial intelligence. The term covers a group of methods that allows software to develop its own algorithms and ‘learn’ how to perform specific tasks without being explicitly programmed to do so. Think of how a toddler learns what the word ‘dog’ means. The child may use all kinds of clues to develop and refine their understanding of ‘dog,’ including the context in which adults say ‘dog’ and the reaction of others when the child uses the word. In essence, machine learning methods combine these decision-making and analysis abilities of the human brain with the large processing power and fast recall of computers.
Immunologists agree that PI is underdiagnosed; IDF’s own surveys show that patients often wait a decade or more for a diagnosis after they start exhibiting symptoms. In some ways, diagnosing PI is a perfect use case for machine learning—there are clearly patterns in symptoms, laboratory tests, and patient history that are regularly missed.
The quest to diagnose PI by harnessing computer power began almost two decades ago with the Study Targeting Recognition of Immune Deficiency and Evaluation (STRIDE) project. STRIDE researchers, led by Dr. Charlotte Cunningham-Rundles, developed a scoring system for patient hospital records based on ICD-9 diagnostic codes. ICD codes, now in their tenth edition, are standardized and used by all healthcare providers in the U.S. for insurance and billing purposes.
Using a set of predefined rules and a predetermined score threshold, the researchers programmed software to score thousands of records. Fifty-nine patients who met the threshold were then tested for PI and 17 (29%) were ultimately diagnosed. The original software did not use machine learning, as the scoring system and the score threshold were set by the researchers. But, STRIDE proved that indicators of PI were indeed hiding in patient records waiting to be found.
In more recent efforts, researchers at Texas Children’s Hospital led by Dr. Nicholas Rider developed PI Prob. It also leverages ICD codes from electronic health records but uses machine learning to distinguish between records belonging to children with PI and records belonging to children without PI.
To build PI Prob, researchers first determined which ICD codes were more frequent in records for more than 1,500 children with confirmed PI diagnoses than in records for a control group of children without PI. These codes were further refined using expert knowledge in immunology. In contrast to the STRIDE project though, the codes were not given predetermined scores by the researchers. Instead, they were used for supervised machine learning.
A subset of machine learning technologies, supervised machine learning uses training datasets where every record has a known classification. The software must ‘learn’ how to correctly classify each record within the training set by adjusting the weight given to different features in the data. In the analogy of learning the word ‘dog,’ this is similar to an adult presenting the child with pictures of dogs mixed in with other animals. The child might learn that four legs, a tail, and overall size are features in the photos that help them correctly identify dogs.
For PI Prob, the training set was drawn from the previously described set of records, and the data features were the refined ICD codes. The software then experimented with how to weigh the codes to develop the best model for classifying the training records correctly. After training, the researchers then tested how well PI Prob could distinguish records it had not seen before. Overall, it was 89% accurate in distinguishing records for children with PI from records for children without PI.
A related study from researchers at the University of Chicago, funded by Takeda, attempted to develop a model that works for both children and adults and expanded on the type of data features the software could consider in order to ‘learn.’ In addition to ICD codes, their software counts how many orders for laboratory tests, radiological tests, and prescriptions for medication meant to manage PI symptoms are in each record. The researchers showed that adding these clinical features improves the accuracy of their algorithm by 7% versus using ICD codes alone.
However, the accuracies of both PI Prob and the University of Chicago tool were tested only on known datasets—that is, records for individuals who were already known to either have a PI or not. The true test is to deploy such a tool proactively and determine if the individuals it flags are ultimately diagnosed with PI, as in the original STRIDE study.
In a paper published in October 2022, researchers, again led by Rider, got one step closer to truly testing machine learning for diagnosing PI. They developed a two-step pipeline using the Jeffrey Model Foundation’s Software for Primary Immunodeficiency Recognition, Intervention, and Tracking (SPIRIT) Analyzer and a machine learning tool that triages high-risk children for immunology referrals based on the percentage of their medical visits that concern PI symptoms. The initial machine learning was done using a training set of records from children with PI diagnoses and from children with secondary or no immunodeficiency diagnoses.
However, the group then deployed the pipeline within the Texas Children’s Health Plan, which is a Medicaid program. Thirty-seven total children were proactively flagged for immunology referrals. Unfortunately, some of the individuals were lost to follow up and the authors did not know the referral outcomes for the rest at the time of publication.
X4 Pharmaceuticals, a company that focuses on chronic neutropenia, has taken a different tact. To align with their focus, they are using machine learning to identify individuals with one specific PI, warts, hypogammaglobulinemia, infections, and myelokathexis (WHIM) syndrome, rather than PIs in general.
To train their machine learning software, X4 used a decades-worth of medical records for 32 individuals with genetically confirmed diagnoses. By asking the software to construct a ‘typical’ medical record for WHIM syndrome and then looking for similar patient records within an insurance claims database, X4 estimates that there are between 1,800-3,700 individuals with WHIM syndrome in the U.S. Even the low estimate is substantially more than current prevalence numbers, which place the number at less than 1,000 individuals with WHIM syndrome living in the U.S. The company has not released information on whether they intend to use the software to identify specific individuals at high risk for a WHIM diagnosis.
Despite these successes, there are challenges ahead in using machine learning software to find individuals with PI. The U.S. healthcare system is fractured over many providers and insurers who all use their own data systems. Designing one tool that can operate across those systems, and convincing providers and insurers to implement it, will be difficult.
In addition, since the tools developed to date rely on training datasets, they may have to be trained and retrained depending on where they are deployed to account for differences in patient populations. After all, if a toddler is given a training set of pictures of dogs mixed in with pictures of birds, their definition of the word ‘dog’ might not hold up when pictures of cats are introduced. In other words, supervised machine learning tools are very sensitive to any biases in their training datasets and must be validated in new contexts.
The studies detailed above are a promising start to using machine learning in the diagnosis of PI. With luck and ingenuity, these tools will come into common clinical use, saving those with undiagnosed PI years of uncertainty.
This page contains general medical and/or legal information that cannot be applied safely to any individual case. Medical and/or legal knowledge and practice can change rapidly. Therefore, this page should not be used as a substitute for professional medical and/or legal advice.
Receive news and helpful resources to your cell phone or inbox. You can change or cancel your subscription at any time.
The Immune Deficiency Foundation improves the diagnosis, treatment, and quality of life for every person affected by primary immunodeficiency.
We foster a community that is connected, engaged, and empowered through advocacy, education, and research.
Combined Charity Campaign | CFC# 66309