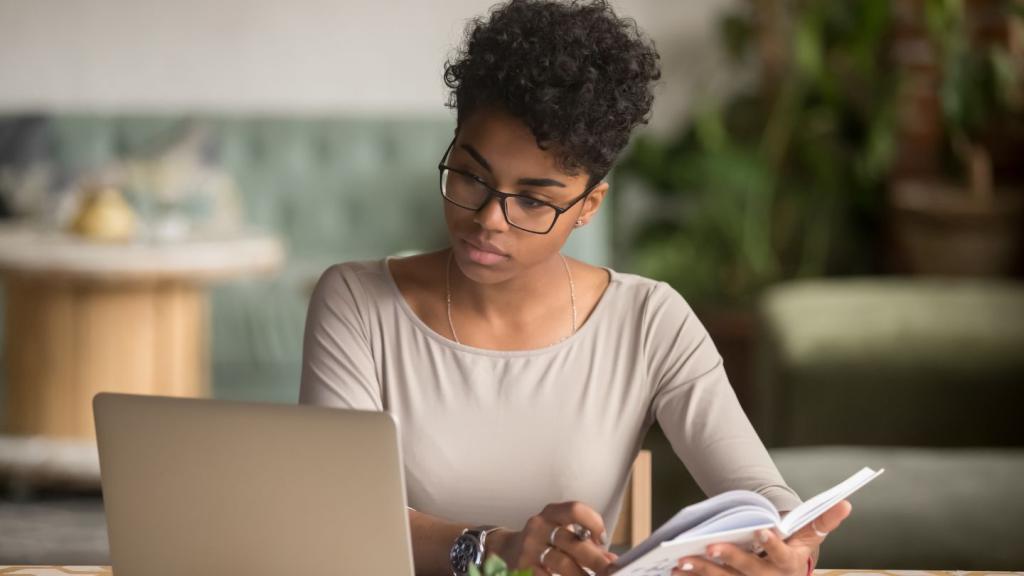
The more you understand about primary immunodeficiency (PI), the better you can live with the disease or support others in your life with PI. Learn more about PI, including the various diagnoses and treatment options.
Living with primary immunodeficiency (PI) can be challenging, but you’re not alone—many people with PI lead full and active lives. With the right support and resources, you can, too.
Be a hero for those with PI. Change lives by promoting primary immunodeficiency (PI) awareness and taking action in your community through advocacy, donating, volunteering, or fundraising.
Whether you’re a clinician, researcher, or an individual with primary immunodeficiency (PI), IDF has resources to help you advance the field. Get details on surveys, grants, and clinical trials.
The Immune Deficiency Foundation (IDF) is proud to fund four research projects totaling more than $150,000 through the 2024 IDF Research Grant Program. The funded projects each work towards reducing the time to diagnosis for those with primary immunodeficiencies (PIs), also known as inborn errors of immunity (IEI), a core goal of the foundation.
As the applicant with the highest-scoring grant, this year’s Michael Blaese Research Grant Award goes to Dr. Emily Harris, a clinical fellow in pediatric hematology/oncology at Dana-Farber/Boston Children’s Cancer and Blood Disorders Center. Harris plans to use the funding to investigate Evans syndrome to develop better diagnostic and treatment tools.
Evans syndrome is a rare and chronic autoimmune condition in which the immune system destroys at least two different types of blood cells—typically platelets and red blood cells. While not a PI itself, in children, Evans syndrome often signals that the person has an underlying PI. Harris and her collaborators recently identified a group of T cells, named cTfm1/17 T cells, that may distinguish Evans syndrome from other types of autoimmune blood cell destruction.
Harris plans to look at cTfm1/17 T cells more closely to see what genes they have turned on or off and whether these gene expression patterns explain what the cells are doing. She hopes her data will also lead to a clinical test to detect cTfm1/17 T cells, which could help clinicians diagnose Evans syndrome sooner.
The second part of Harris’ project focuses on another gap in caring for those with Evans syndrome—determining which treatment will be effective. As with other kinds of autoimmunity, treating Evans syndrome involves broadly suppressing the immune system with medications like steroids, rituximab, cyclosporine, sirolimus, and others. However, clinicians don’t know who will respond to which medication and must currently rely on their own experience and trial and error to find the regimen that works for each patient.
To address this gap, Harris plans to use stored blood samples from patients with Evans syndrome to test whether medications that lower the percentage of cTfm1/17 T cells in the lab match those that were used to successfully treat each patient. If so, cTfm1/17 T cells could form the basis of not only a diagnostic test but also a test that helps clinicians choose the right treatment for their patients.
“Our goal is to study the immune systems of patients and then leverage this knowledge to not only find a diagnostic answer but also to predict which treatments are most likely to be effective,” Harris said.
Grantee Dr. David Nguyen, an assistant professor at the University of California, San Francisco, wants to determine if genetic variants in the JAK3 gene cause the immune system problems seen in four patients whose symptoms of immune dysregulation remain genetically unexplained. Researchers already know that people with certain variants in both copies of JAK3 have severe combined immunodeficiency (SCID) because their cells don’t have any functional JAK3 protein. JAK3 protein helps cells respond internally to outside chemical signals. It’s particularly important for the development of T cells, B cells, and natural killer (NK) cells.
The four patients of interest have less severe immunodeficiency, plus autoimmune symptoms, and their JAK3 variants only occur in one of their two copies of the gene. Nguyen thinks these individuals may have a new type of PI caused by dominant JAK3 variants that have not been described before.
“We hope to better understand JAK3 mutations to achieve a molecular diagnosis for our patients, but in doing so, we could also be helping the [PI] community understand many other poorly understood [PIs],” Nguyen said.
To test his hypothesis, Nguyen plans to use a new type of CRISPR gene editing technology he helped develop that puts less stress on cells to re-create the variants these patients have in T cells, B cells, and NK cells donated by people who do not have PI. By testing the edited cells to see how each variant affects cell signaling and which genes are turned on and off, Nguyen hopes to find strong evidence linking these variants to each patient’s symptoms.
“This IDF funding will catalyze the application of these new gentle editing technologies to assessing JAK3 mutations,” Nguyen explained. “If successful, these tools can be easily applied to help recognize and diagnose other [PI] genes and disease states, and ultimately will pave the way for developing gentle CRISPR editing of patients’ cells as therapeutic strategy to correct [PI] mutations.”
One of the issues that has hampered the use of machine learning in PI diagnosis is the need to flag a predetermined set of patient characteristics, like diagnostic codes or test results, as important ‘features’ for the algorithms. The algorithms then use these features to figure out how to accurately classify a set of training data, where every patient record already has a known classification (i.e., PI diagnosis or no PI diagnosis). The problem with this approach is two-fold: human experts have to decide which features are important for diagnosing PI and the process relies heavily on how representative the training records are of the patient data the algorithm will eventually analyze.
Dr. Alexandra Martinson, a pediatrics research track resident at Children's National Hospital in Washington, D.C., plans to take the next leap in machine learning for PI diagnosis. Instead of picking features, she will use unsupervised clustering methods on a database of millions of anonymized electronic health records (EHRs). The algorithm won’t know ahead of time which records belong to those with a PI diagnosis or which characteristics to pay attention to, which will allow it to discover patterns in the data without the input, and potential bias, of human experts.
Martinson hopes that clustering, which has been used in medical imaging analysis, will reveal features that haven’t been considered before in machine learning to diagnose PI. “The pattern recognition algorithm will allow for earlier detection of IEIs through systematic recognition of disease-defining features on a multicenter, national big data set,” she said on the impact of her work.
The use of a big data set drawn from more than a hundred medical centers is also a leap forward. Martinson anticipates that there will be enough data from people with different types of PI to determine if there are features specific for subsets of PI, like antibody deficiencies or combined immunodeficiencies.
Ultimately, Martinson plans to incorporate features her unsupervised machine learning finds into the Jeffrey Model Foundation’s Software for Primary Immunodeficiency Recognition, Intervention, and Tracking (SPIRIT) Analyzer. Doing so will improve a tool that’s already known and in use in the PI community.
Dr. Lauren Meyer, a pediatric hematology/oncology fellow at Seattle Children’s Hospital, is focused on hemophagocytic lymphohistiocytosis (HLH). HLH happens when certain cells of the immune system become overactive and cause widespread inflammation. Symptoms can include a high fever, rash, liver inflammation, jaundice, and low blood cell counts, which can progress to organ damage and death if not treated promptly. Sometimes, HLH is a symptom of another condition like cancer or chronic granulomatous disease (CGD), but it can also be a PI itself with no other underlying cause.
Diagnosing HLH is difficult because its symptoms overlap with other, more common conditions and the available diagnostic algorithms include specialized laboratory tests that are not widely available.
“We know that patients who are diagnosed quickly and started on medications earlier in their disease course have a better chance of survival, but diagnosing HLH can be very challenging,” Meyer said.
In previous work, Meyer and her colleagues identified a trio of commonly available laboratory tests that can distinguish patients with HLH from those without the condition. The tests measure blood levels of two proteins, ferritin, which stores iron inside of cells, and fibrinogen, which helps form blood clots, as well as triglycerides, a type of fat. Meyer will use her grant funding to further develop a screening tool for HLH by determining the appropriate cutoff values for these three tests.
Said Meyer about receiving funding, “Support from IDF will allow us to collect data on a larger patient population, ensuring that our results are broadly generalizable. This support will also allow us to perform more extensive data modeling in order to optimize our diagnostic tool and will facilitate prospective validation and future implementation of this diagnostic strategy.”
Missed a session or want a refresher? 2024 PI Conference session recordings are now available!
Browse sessionsThis page contains general medical and/or legal information that cannot be applied safely to any individual case. Medical and/or legal knowledge and practice can change rapidly. Therefore, this page should not be used as a substitute for professional medical and/or legal advice. Additionally, links to other resources and websites are shared for informational purposes only and should not be considered an endorsement by the Immune Deficiency Foundation.
Receive news and helpful resources to your cell phone or inbox. You can change or cancel your subscription at any time.
The Immune Deficiency Foundation improves the diagnosis, treatment, and quality of life for every person affected by primary immunodeficiency.
We foster a community that is connected, engaged, and empowered through advocacy, education, and research.
Combined Charity Campaign | CFC# 66309